P709 Distinct Pattern of Gut Microbial Dysbiosis in Crohn's Disease and Intestinal Tuberculosis - A Machine Learning-based classification model
Markandey, M.(1);Bajaj, A.(1);Vuyyuru, S.K.(1);Mohta, S.(1);Singh, M.(1);Verma, M.(1);Kumar, S.(1);Kante, B.(1);Kumar, P.(1);Makharia, G.(1);Kedia, S.(1);Ahuja, V.(1);
(1)All India Institute of Medical Sciences, Department of Gastroenterology and Human Nutrition, New Delhi, India;
Background
Crohn’s disease (CD) and intestinal tuberculosis (ITB) are chronic granulomatous inflammatory disorders characterized by a compromised mucosal immunity. Even with diverging etiologies, CD and ITB presents an uncanny resemblance in clinical manifestation resulting in diagnostic dilemma. The gut microbiota regulates myriad of gut mucosal immunological processes. Present study aims to decipher gut microbial dysbiosis in the two disorders and utilize the CD and ITB-specific gut dysbiosis to construct a machine learning (ML)-based predictive model, which can aid in their differential diagnosis.
Methods
Fecal samples from healthy controls (n=12) and from patients with CD (n=23) and ITB (n=25) were subjected to 16S (V3-V4) amplicon sequencing. Processing of raw reads, construction of ASV feature tables, diversity, core microbiome analysis and ML classifier construction was done using QIIME2-2021.4. Differential abundance analysis (DAA) between the groups was carried out using Deseq2, after adjusting for the subject-specific confounders.
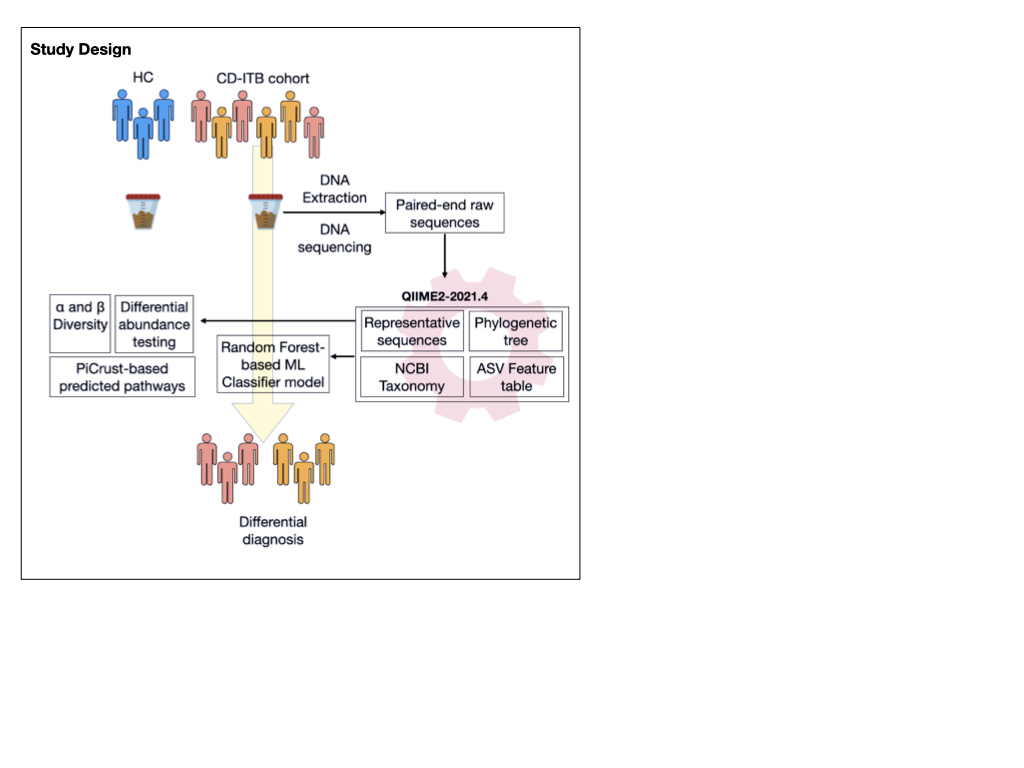
Results
The α and β diversity indices in CD and ITB groups were significantly reduced than HC group (p = 0.011 and 0.012 resp.), with no significant differences between the two diseases (Fig.1A, 1B). On comparison with HC, CD and ITB groups showed reduction in members of Firmicutes and Bacteroidetes, with enhancement of Actinobacteria and Proteobacteria (Fig.1C and 1D). DAA (FDR q <0.1, FC >2.5) between CD and ITB groups revealed expansion of Succinivibrio dextrinisolvens, Odoribacter splanchnicus, Megasphaera massiliensis, Bacteroides uniformis and B.xylanisolvens in CD group, while Clostridium sp., Haemophilus parainfluenzae and Bifidobacterium sp. were elevated in ITB (Fig.2A). Random Forest-based ML model constructed on the basis of raw microbiome reads and using 80% of the samples to train the model, showed predictive accuracy of 0.78 (AUC=93%). (Fig.2B)
Conclusion
Our study shows that CD and ITB witnesses significant changes in gut microbial structure. With no significant differences in microbial diversity between two diseases, the signature of gut dysbiosis is distinct between CD and ITB. Exploitation of these differences to construct ML models can potentiate differential diagnosis of CD and ITB.
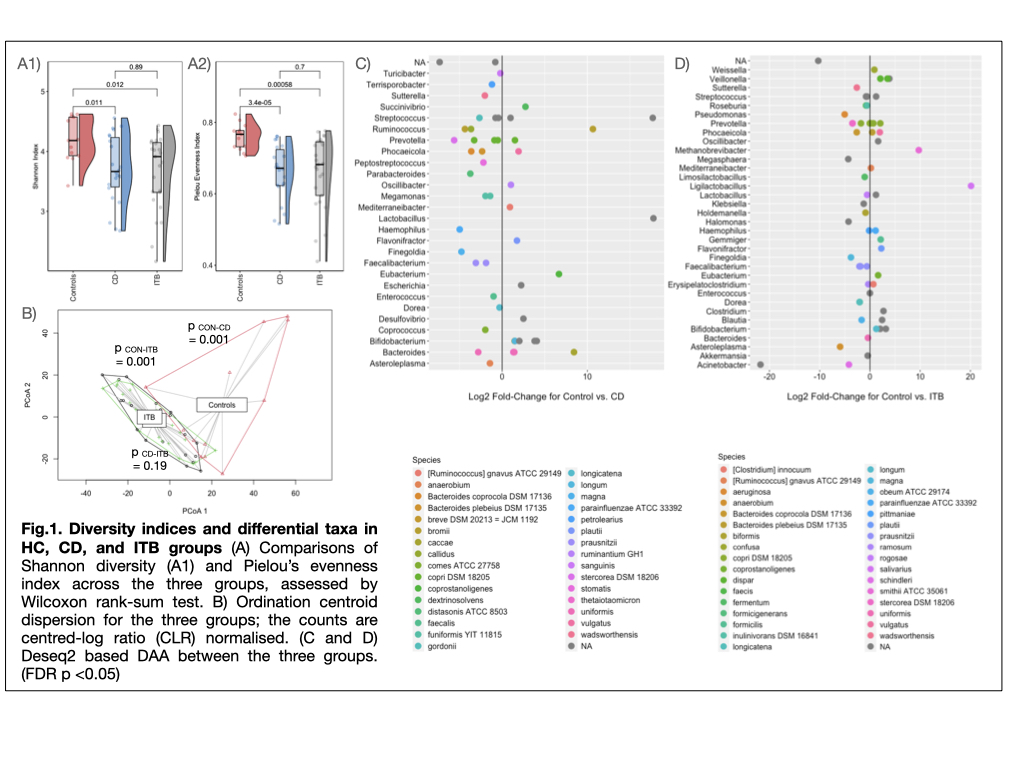
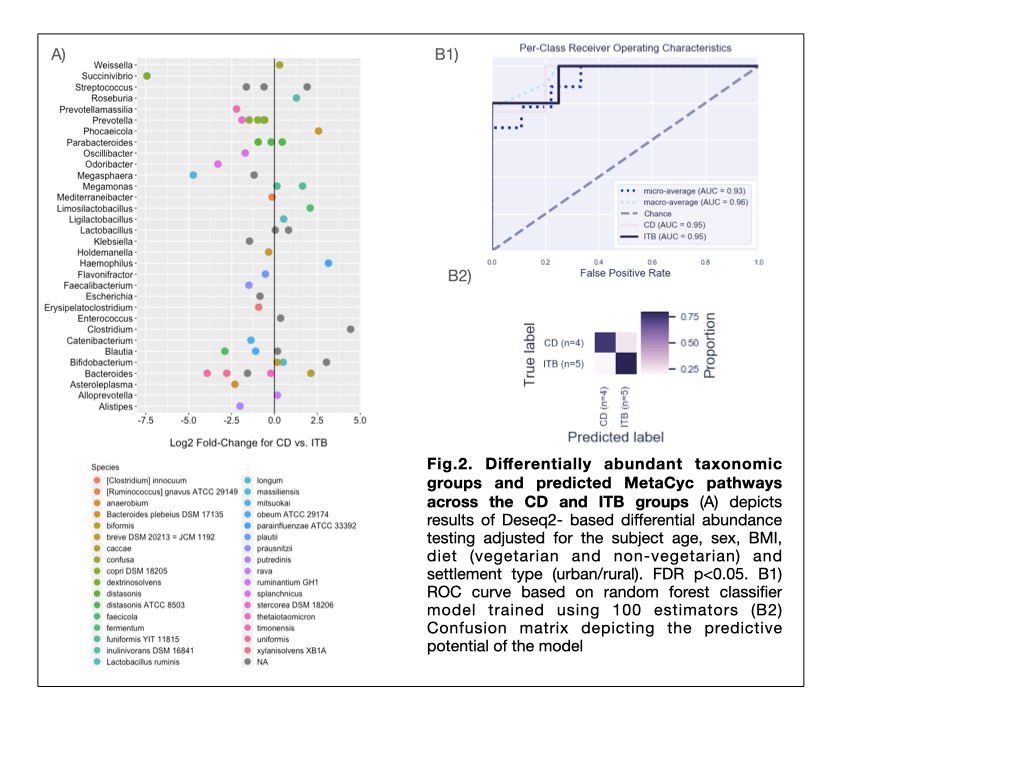